- The Context Window
- Posts
- Multi-Modal AI
Multi-Modal AI
Your Quick Guide to AI That Reads, Sees, Hears & Thinks (Maybe) 😉
Chances are, if you’ve spent any time playing around with AI, you’ve heard the term Multi-modal AI—maybe even experienced it or used it without realizing. So what exactly is it, why does it matter, and why is it kind of a big deal? 🤔
What Exactly Is Multi-Modal AI?
In plain English: Multi-modal AI is artificial intelligence that doesn’t just stick to one type of information. It can simultaneously understand, interpret, and even generate content using a mix of:
Text: The words you’re reading now, reports, emails, code.
Images: Photos, diagrams, charts, that weird meme your intern sent.
Audio: Spoken language, music, sound effects, your CEO’s slightly-too-loud phone calls.
Video: Presentations, product demos, security footage.
And sometimes even other data like sensor readings or 3D models.
Think of it like this: A traditional AI might read a document. A multi-modal AI can read the document, look at the accompanying images, listen to a voice note explaining a key chart, and then give you a summary that considers all of it. It’s about connecting the dots between different kinds of data to get a richer, more contextual understanding – much like you do every day without thinking about it.
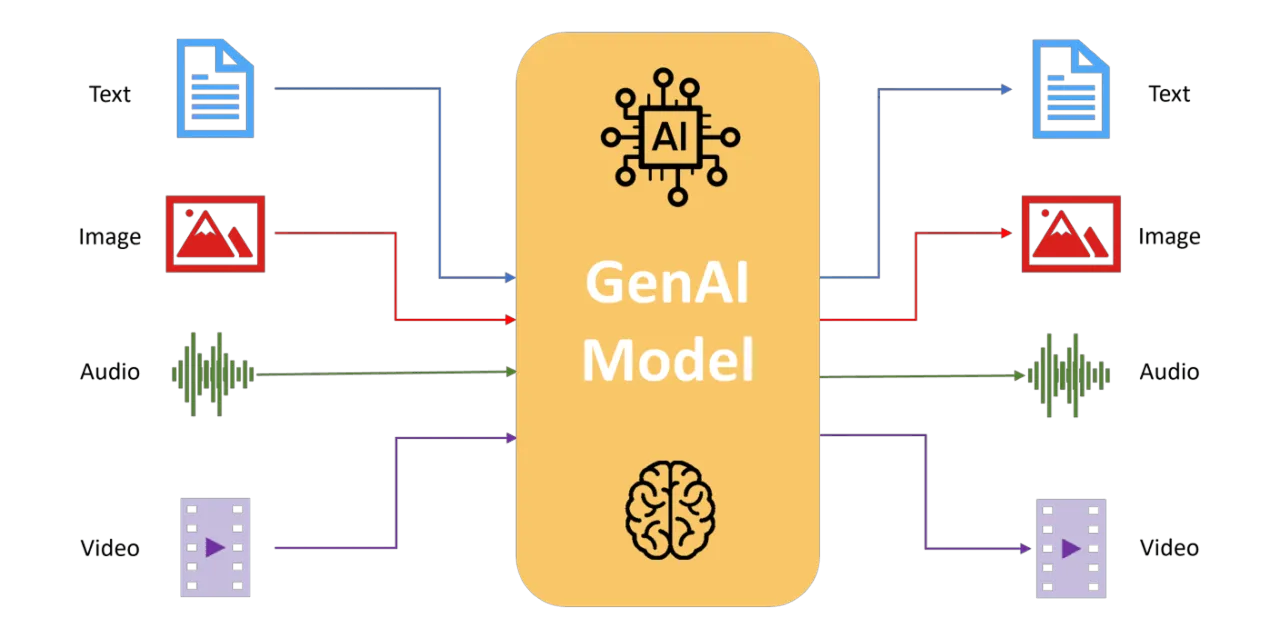
Multi-modal AI integrates diverse data types like text, images, audio, and video. Source: Ultralytics (2025)
Why Should You, a Busy Leader, Actually Care?
So Multi-modal AI sounds cool, right? But why does it matter?
➡️ Deeper Insights from Your Data: Your business already has tons of data in different formats. Multi-modal AI can help unlock insights you’re currently missing by analyzing these varied sources together.
💡 Imagine understanding customer sentiment not just from survey text, but by correlating it with product images they share or the tone of their voice in support calls.
➡️ More Natural & Efficient Human-AI Interaction: Instead of just typing, you can talk to an AI, show it things, or have it watch a process. This makes AI more accessible and potentially much faster for certain tasks. OpenAI’s GPT-4o (or later) or Google’s Gemini, which are designed for these more fluid, multi-sensory interactions.
➡️ Automation of Complex Tasks: Tasks that previously required human interpretation of multiple data types can now be automated. This could be anything from analyzing complex insurance claims (photos, reports, adjuster notes) to monitoring a manufacturing line (video feeds, sensor data, audio alerts).
➡️ New Product & Service Possibilities: Multi-modal capabilities can unlock entirely new applications – from hyper-personalized retail experiences to advanced accessibility tools for people with disabilities.
Essentially, it’s about making AI more versatile, more context-aware, and ultimately, more useful in solving real-world business problems.
What Can It Do In The Real-World?
Let’s get practical. What are some practical use cases for this?
✅ Enhanced Customer Service: Imagine being able to analyze customer support calls more comprehensively: AI analyzing a customer’s voice tone, facial expression (in video calls), and chat history to provide more empathetic and effective support.
✅ Smarter Content Creation: Generating marketing copy based on a product image, target audience profile (text), and a desired mood (e.g., from a reference audio clip). Tools like GPT-4o are heading this way.
✅ Intelligent Document Processing: Extracting information from complex documents that mix text, tables, and images (like invoices or legal contracts) with much higher accuracy.
✅ Retail & E-commerce: Amazon’s StyleSnap lets users upload a photo of an outfit they like, and the AI finds similar items. This combines image understanding with product catalog data (text and images).
✅ Healthcare Diagnostics: AI assisting doctors by analyzing medical images (X-rays, scans) alongside patient notes (text) and lab results (data) to help spot patterns or anomalies.
⚠️ As with all AI aspiration - this one’s got some more maturing to do.
✅ Accessibility Tools: Real-time image description for the visually impaired, or converting speech to sign language avatars.
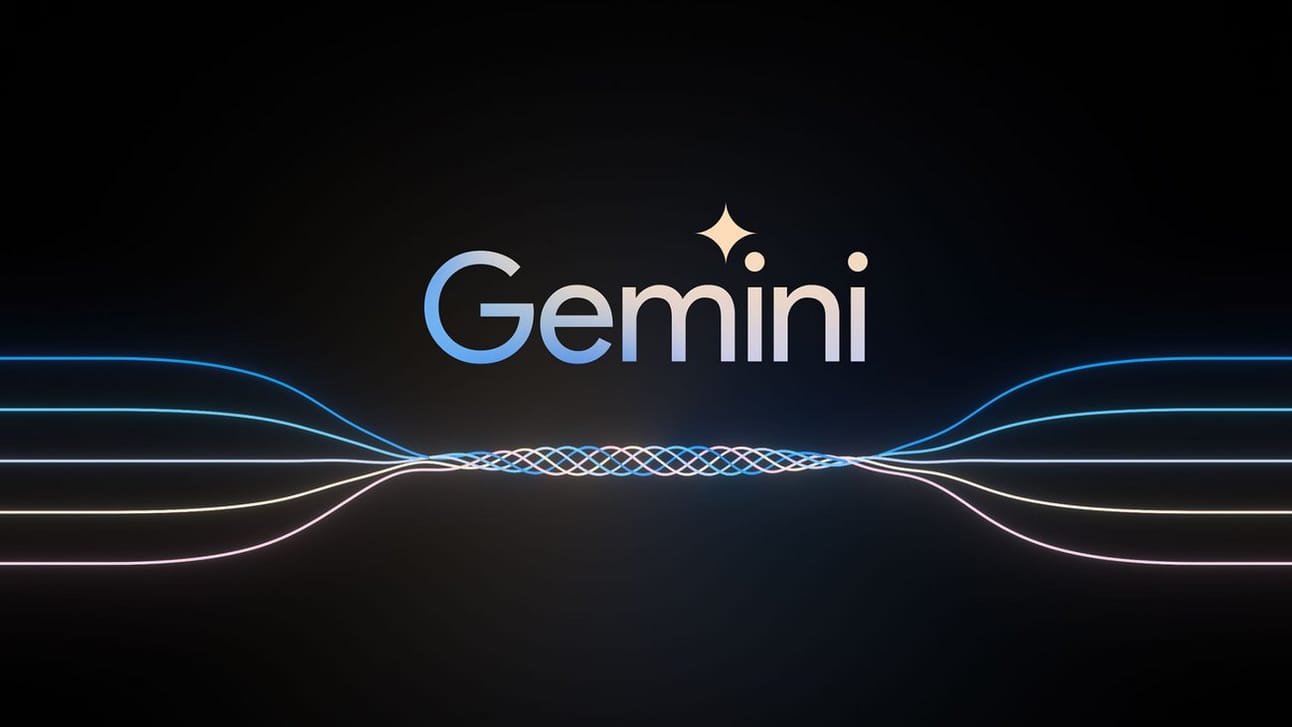
Google's Gemini is an example of a natively multimodal AI model. Source: Google
The Not-So-Pretty Picture: Risks & What to Watch For
As with all AI innovation - this isn’t a silver bullet. It comes with its own set of headaches, and as a leader, you need to be aware of them:
⚠️ Increased Complexity & Cost: Training and running these models can be significantly more resource-intensive than text-only versions. Which means if your business wants to use them, there is a higher cost factor. The good news is - the cost of AI models across the board are dropping by the day.
⚠️ The “Garbage In, Garbage Out” Problem, Magnified: If your image data is biased, or your audio data is poor quality, the AI’s multi-modal insights will be flawed. The quality of all input data types is critical.
⚠️ Deepfakes & Disinformation on Steroids: The ability to generate convincing fake video, audio, and text together makes creating sophisticated disinformation much easier. The Enkrypt AI report on Mistral’s Pixtral (May 2025) highlighted how these models can still be jailbroken to create harmful or misleading content, even with images involved.
⚠️ Bias Amplification: Biases present in one data type (e.g., text) can be reinforced or even amplified when combined with other data types. An AI might learn to associate certain visual features with negative sentiment in text, leading to biased interpretations.
⚠️ Data Privacy Concerns: Handling multiple types of sensitive personal data (voice, images of faces, etc.) raises significant privacy and security challenges. Who owns this combined data? How is it protected?
⚠️ Explainability (The Black Box Problem): If it’s hard to understand how a text-AI made a decision, it’s even harder when it’s juggling images, audio, and text. Understanding why a multi-modal AI reached a conclusion can be a nightmare.
Final Thoughts
Multi-modal AI is truly a fundamental shift towards AI that interacts with information in a more human-like way. It’s here to stay and getting so much better every day.
When Multi-modal was first launched the interpretation of audio and output of was a 2/10 on a good day, but only a year down the line and it’s getting hard to tell what’s AI generated and what’s not.
This is very exciting but also you need to exercise good judgement.
The bottom line: Start exploring, but do it with your eyes wide open and a healthy dose of skepticism. Understand the capabilities, but more importantly, understand the limitations and the risks. This isn’t about replacing humans; it’s about augmenting them.
Want to learn more about Multi-Modal AI? Reply to this email or drop a comment on X (@hashisiva).
💡 We are out of tokens for this week’s Context Window!
Thanks for reading!
Follow the author: X at @hashisiva | LinkedIn |
How helpful was this week's email? |